How and why to use Key Drivers Analysis in user research
A step-by-step approach to understanding what’s behind customer sentiment and behavior
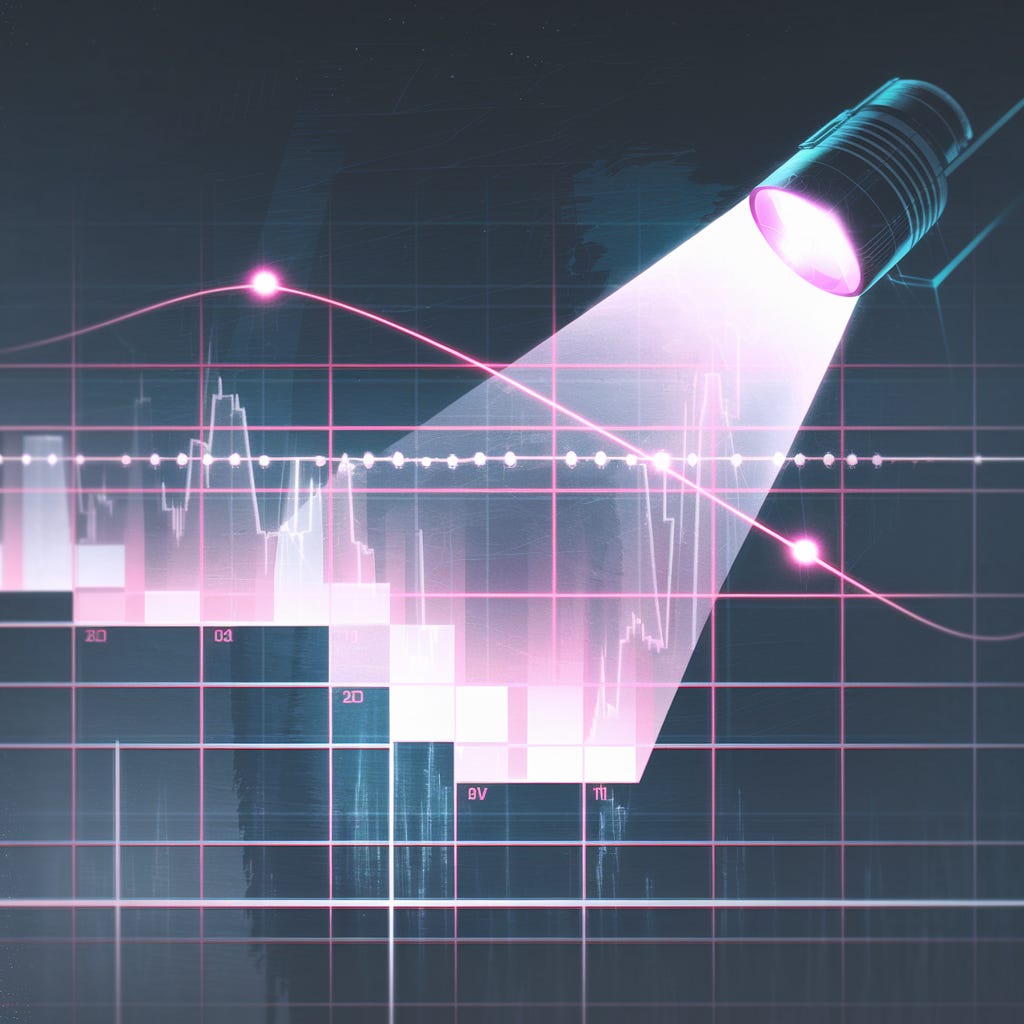
In just about any context, human behavior and decision-making can be complex, and it rarely hinges upon a single factor.
Consider something as seemingly straightforward as purchase intent. Is it all about the price? Does easy website navigation influence the decision? Or maybe a product feature stands out? Most likely, it’s a blend of these and other factors. Without a clear understanding, it’s easy to waste resources, focusing on aspects that may not significantly impact user behavior.
Key Drivers Analysis (KDA) is a specific technique that quantifies the elements of your product or service that most impact outcomes like purchases, satisfaction, or retention. By quantifying the influence of each factor, it takes the guesswork out of deciding where to focus development, marketing, and sales resources.
In this article, we’ll introduce KDA, explain its purpose, and compare and contrast it with similar methods. You’ll learn how to conduct a KDA, and how to visualize and interpret the results. By the end, you’ll add KDA as an essential tool in your toolkit for making informed, data-driven decisions.
What is a Key Drivers Analysis?
KDA aims to uncover the independent variables—called drivers—that most strongly influence a desired outcome.
In product development, this means identifying which features or attributes have the greatest impact on key performance indicators like customer satisfaction, perceived effort, purchase intent, churn likelihood, or loyalty. KDA helps answer essential questions such as:
What drives users’ perception of trust while using our website or app? Is it the content, usability, or loading speed that matters most?
What factors influence the decision to purchase or subscribe? Is it price, product features, or the quality of customer support?
The concept behind KDA is straightforward. Imagine you want to predict whether customers will recommend your product. Your team is aware of several potential factors—such as price, product quality, or ease of use—that could influence their decision. KDA uses statistical techniques, often through a method called multiple linear regression, to analyze these factors and determine which are most influential. In simple terms, KDA reveals how much each factor impacts the overall outcome, which gives teams much more confidence in their product decisions.
For those less familiar with quantitative methods, KDA may seem similar to MaxDiff and conjoint, which are also popular for measuring customer preferences. However, each method serves a distinct purpose, addressing related but unique questions. KDA’s strength lies in its ability to quantify how much each factor influences a specific outcome. Here’s a quick comparison with these other two common methods:
By understanding these differences, you can choose the right method—or combination of methods—to gain the clearest insights into what drives your customers and where to focus your efforts.
How to conduct a Key Drivers Analysis
A KDA may sound complex, but breaking it down into simple steps can make it manageable, provided that you have some confidence with inferential statistics. Here’s an overview of how KDA is done:
1. Define the outcome variable and potential driver variables
Start by selecting a single outcome variable (e.g., customer satisfaction or purchase intent) and potential driver variables (e.g., product features or usability factors) that your company has some influence over. Your choices should align with business objectives and be based on prior research efforts (if you have any), or at least, solid theoretical grounding. At a high level, that means making sure the predictors logically precede or influence the outcome. Also avoid any obviously irrelevant variables to prevent noise from clouding your results.
2. Collect data
The next step is to collect data that includes both outcome and driver variables. While this can come from a range of sources—such as unmoderated UX benchmarks, intercept surveys, or live product analytics—often, the best approach is to design a Key Drivers survey specifically for this purpose. For example, if your outcome variable is Customer Satisfaction (CSAT), you’ll ask the standard CSAT question alongside other questions targeting attitudes and behaviors that may influence satisfaction.
A note on sample size: the ideal target will depend on factors such as the number of drivers you’re assessing, the expected strength of their impact, and whether you’re comparing different participant groups. Generally, sample sizes between 50 and 150 are common for meaningful results.
3. Prepare a statistical tool for the analysis
Once your data is collected and cleaned, import it into a robust statistical tool. Options include commercial packages like SPSS or SAS, or free tools like R, Python, JASP, or Jamovi. These tools allow you to process the data and run the necessary calculations to determine key drivers.
4. Ensure data meets assumptions
Review the data for potential issues like collinearity, non-linear relationships, or homoscedasticity.
Although a full treatment of these considerations is beyond the scope of this overview, we can consider collinearity, when two or more factors are closely related, as an example. This common issue can threaten any meaningful interpretation of your results — ideally, you would head this off in Step 1 by defining clearly distinct variables. Nevertheless, there are a few statistical approaches to help you identify or avoid multicollinearity problems, such as by calculating the Variance Inflation Factor (VIF; values above 10 typically indicate a problem), or averaging over orderings.
5. Calculate the importance of each factor
In most cases, you will next run a multiple linear regression analysis. This step identifies how different factors relate to your outcome and, specifically, which factors have the most significant influence.
Calculate how much of the outcome your factors explain and how much remains unexplained. Use standardized beta weights to express each factor’s influence in comparable units. Then, use the R-squared value (or proportion of total variance explained) to convert these weights into percentages to rank which factors matter most.
6. Visualize and apply the insights
Finally, generate visualizations to bring your results to life. Visuals will help illustrate which factors are most important and how they compare with each other. In the next section, we’ll explore common visualizations for presenting KDA results and discuss how to use these insights to inform better business decisions.
What does a Key Drivers output look like?
The output of a KDA may be presented through a variety of visuals, each offering a unique perspective on the data.
One of the most common and effective ways to display results is a scatter plot combined with a 2×2 matrix. In this figure, each factor is plotted along two key dimensions: importance (how much it influences the outcome) and performance (how well the factor is currently doing). The visual is then divided into four quadrants:
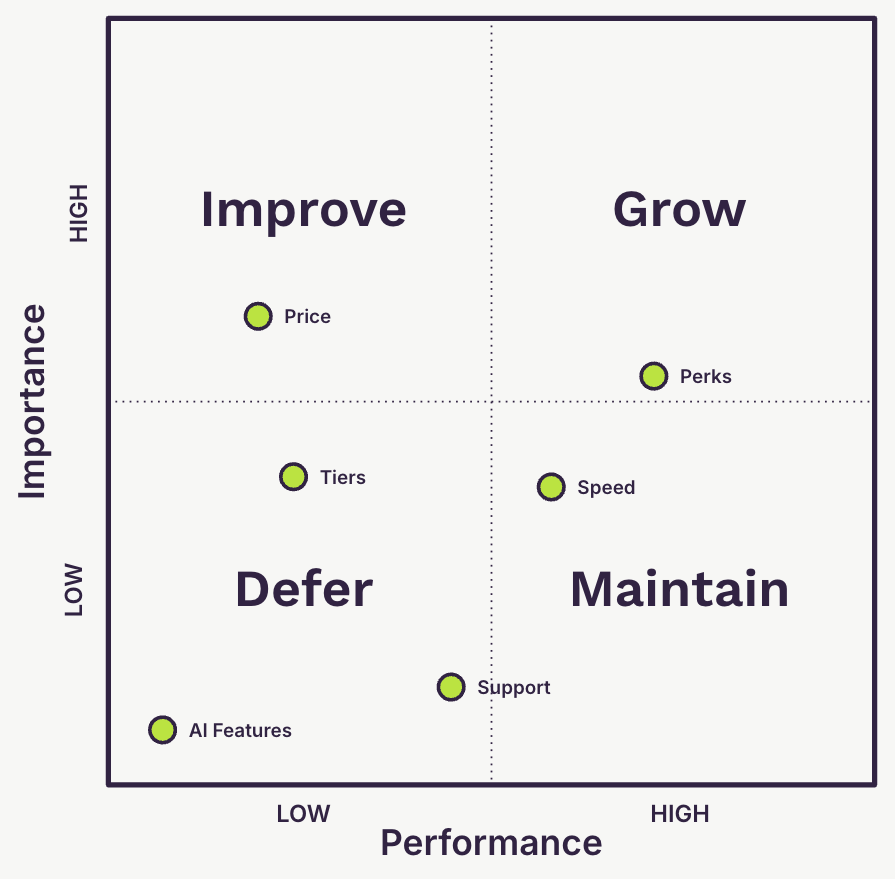
Improve (high importance, low performance): These are critical areas that need urgent attention because they have a significant impact on the desired outcome but are currently underperforming.
Grow (high importance, high performance): These are your strengths, and you should further capitalize on them.
Maintain (low importance, high performance): These areas are performing well but have a lower impact, so you can focus efforts on keeping them where they are.
Defer (low importance, low performance): These factors matter least, and so should be the lowest priority for investment.
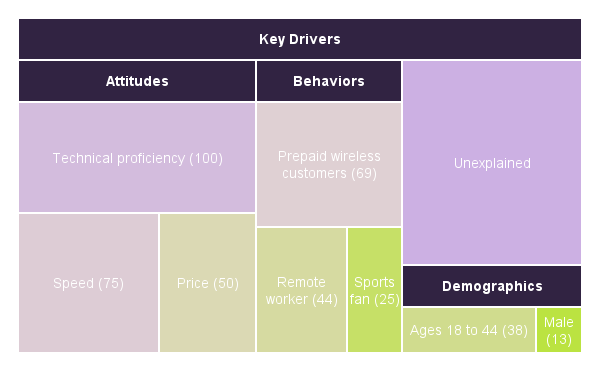
Another visual output to consider is a proportion chart. This rectangular chart, resembling a mix between a pie chart and a treemap, visually represents the relative contribution of each key factor as a score or percentage of the overall variance in the outcome. Each “slice” or section of the chart illustrates how much of the outcome variability is explained by each driver.
One feature that might stand out in this chart is the unexplained variance. This reflects the portion of the outcome that cannot be explained by the factors in your current model. Even the best models will leave a significant amount of variance unexplained, as human behavior and customer decisions are often influenced by unpredictable or unmeasured factors. You’ll need to use your discretion when discussing unexplained variance with non-researchers, however, as this could backfire and undermine the credibility of your results.
Substantial unexplained variance can also suggest that there are other important drivers missing from the current analysis or that more data is needed to better capture customer behavior—an opportunity for further exploration.
The bottom line
Understanding what drives your customers’ behaviors and judgments can better inform important business decisions. Key Drivers Analysis (KDA) helps you identify and quantify those most influential factors, allowing you to focus resources on what truly matters.
KDA uncovers the most influential drivers behind outcomes like customer loyalty, purchase decisions, or service interactions.
Compared with other methods like conjoint and MaxDiff, KDA provides a precise measure of influence, highlighting not only what matters but how much it matters.
Conducting a KDA involves defining outcome and driver variables, collecting and cleaning data, running multiple linear regression, and developing visual outputs that inform.
Visualizations such as scatter plots/2×2 matrices, and proportion charts provide a clear picture of which areas to improve, grow, maintain, or deprioritize.
KDA equips you with actionable insights that guide where to focus your efforts for maximum return—whether that means refining your product features, improving trust, or boosting customer satisfaction.
Drill deeper
These are the broad considerations to keep in mind when conducting your first KDA—but as always, your specific context might have additional challenges. If you’re looking for a partner to help overcome those obstacles or make sure you’re running the right analyses, Drill Bit Labs offers the expertise you need.
Whether you need guidance on specific methods or broader strategic support, we’re here to help you achieve your goals. How we help: user research projects to inform confident design decisions and optimize digital experiences, live training courses that teach teams user research skills, including hands-on practice with KDA, and advisory services to improve UX processes and strategy.
Connect with us to discuss your upcoming projects or ongoing UX needs.