Using signal detection theory in UX research
Understanding user errors in finding the right information
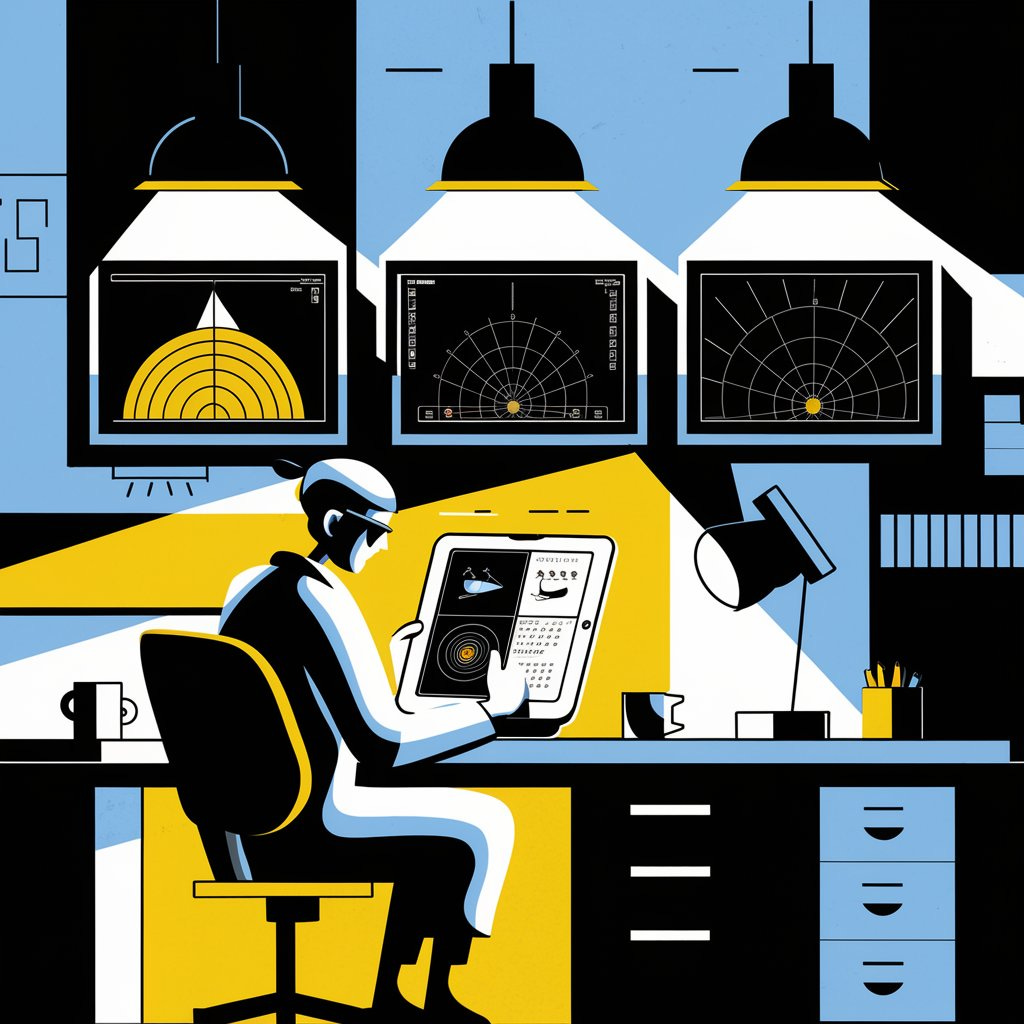
Is that email spam or legitimate? Should that alert be addressed immediately, or can it wait? Is that follower on social media a real person or just another bot?
In everyday situations like these and many others, users make judgments about the information presented to them. While traditional user research methods reveal the challenges users face, they often fall short in capturing the subtleties of these decision-making processes. A simple success rate can’t tell us whether users are effectively filtering out distractions to find what they need or if critical information is getting lost in the noise.
This is where signal detection theory (SDT) comes in. Borrowed from psychology, SDT offers a framework for understanding how users distinguish valuable information from irrelevant distractions. In this article, we’ll look at the core principles of SDT, showcasing its relevance through examples both within UX and in broader contexts. We’ll also provide practical guidance on how to integrate SDT into your user research, helping you gain a deeper understanding of user decision-making.
By the end of this article, you’ll have a solid grasp of SDT and tools to apply it in your future research, enabling you to more accurately assess whether users are finding the information they need in your designs.
The basics of signal detection theory
SDT is a framework introduced by Peterson and colleagues in 1954 to address a common challenge: reasoning and decision-making most often happens under conditions of uncertainty. SDT offers a precise way to dissect and understand how certain decisions are made when the outcomes are not clear-cut. Though first applied in sensory experiments and expanded to other contexts like human factors psychology, the principles of SDT have far-reaching applications, including in UX design and research.
To get a sense of the fundamentals, consider a radiologist examining a CT scan for signs of a tumor amidst normal tissue. Radiologist must make a binary decision: either a tumor is present (signal) or it is not (no signal). They train extensively to gain expertise in this complex task, but their judgments are still imperfect.
This decision-making process results in four possible outcomes:
Hits: When a tumor is present and the radiologist correctly identifies it; i.e., a true signal was successfully detected.
False Alarms: When no tumor is present, but the radiologist mistakenly identifies one. When signals are falsely identified like this, it can cause distress or anxiety, or even prompt unnecessary action.
Misses: When a tumor is present, but the radiologist fails to detect it. At times like this, if a signal is missed, it can lead to serious consequences.
Correct Rejections: When no tumor is present, and the radiologist accurately describes an absence. This reflects a correct decision where no signal is present, so nothing unneeded is undertaken in response.
Real-world examples of SDT
In radiology and many other contexts, hits and correct rejections are the desired outcomes, while misses and false alarms represent errors that can have significant implications. And there are many examples from other fields that may be familiar.
Radar operators during World War II faced a daunting task of interpreting simple circular displays, where fleeting green dots appeared on the screen. These dots could represent enemy aircraft (signals) or noise caused by environmental factors or the radar system itself. The operator had to make a decision: should they alert the nearest air force base for potential enemy interception, or dismiss the blip as noise?
Modern airport security screenings are a more contemporary example of SDT in action. In the US, for instance, TSA officers must rapidly evaluate X-ray images of baggage, distinguishing between harmless items and potential threats like weapons or explosives. Everyday objects can often resemble dangerous items on the screen, creating noise that complicates the identification process. Officers must make swift decisions on whether to flag a bag for further inspection, balancing the need to catch true threats (signals) while minimizing false alarms that could lead to unnecessary delays or panic.
Traditional statistical hypothesis testing has some parallels with SDT. In both, a criterion is established—a threshold that determines the outcome. In hypothesis testing, this criterion is the critical value at which we decide to reject the null hypothesis, indicating that an effect is present (akin to a hit in SDT). SDT requires balancing the risks of false alarms and misses; similarly, hypothesis testing involves managing the trade-off between Type I (false positive) and Type II (false negative) errors.
Similarly, in UX, there are many contexts where users must make judgments based on the information presented to them. Consider:
Distinguishing malicious behavior and misinformation: One common scenario familiar to most internet users we’ve already discussed: identifying whether an email is spam or a phishing attempt. SDT has been effectively applied here, helping systems and users distinguish between legitimate and malicious messages. As AI-generated content becomes more prevalent, SDT may also play a role in detecting deliberate misinformation or manipulation on social media platforms, where discerning truth from falsehood is increasingly challenging.
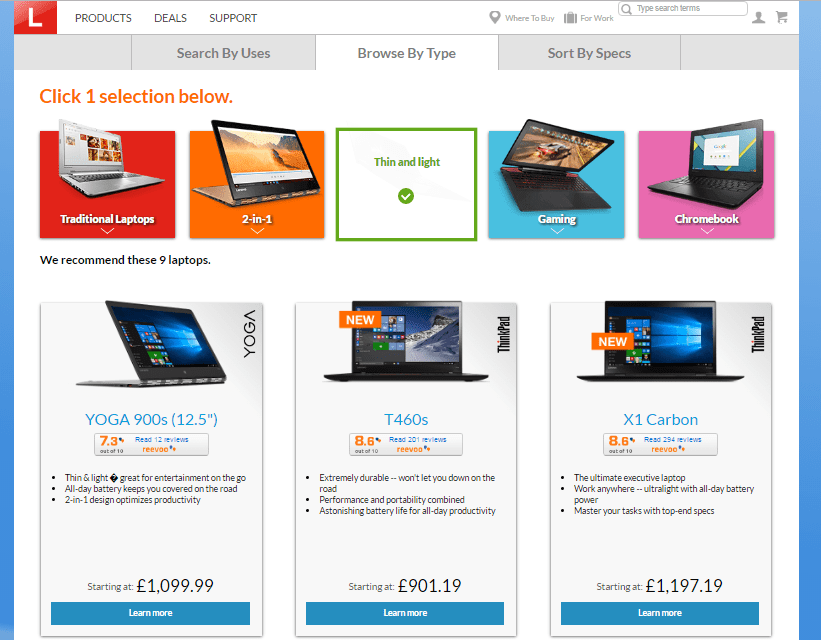
User sensitivity to product signals: Users often seek specific information within product interfaces, and teams want to know if they can find it. For instance, in a clever case study on Lenovo’s e-commerce site, SDT was applied to user data following a redesign that made product detail pages more consistent.
Validity of UX research methods: UX teams working on the cutting edge of emerging technologies sometimes need to use unorthodox or adapted research methods that haven’t been fully validated. SDT can help confirm whether these methods are identifying real opportunities worth pursuing or flagging phantom problems that don’t actually exist, ensuring that the research is both reliable and actionable.
Understanding the fundamental principles of SDT and recognizing situations where it applies is necessary to apply this framework in UX research.
Applying SDT in UX research
How can you effectively use SDT in your next UX research project? The key is to assess whether this framework aligns with your project’s goals and the types of decisions your users need to make.
Begin by determining if your study involves a binary decision—essentially, identifying the presence or absence of a signal, much like in radar detection or security screenings. Once you’ve established this, you can decide how deeply you want to incorporate SDT into your research design and analysis.
At a basic level, you can use traditional UX research methods, such as usability testing, and then overlay SDT concepts to help interpret the data. By categorizing user data into the matrix of hits, misses, false alarms, and correct rejections, you’ll gain a more nuanced understanding of where and why they’re making errors.
For example, if users frequently overlook an important alert during testing, you could map these instances to the matrix to identify patterns of misses and false alarms. This approach may reveal more specific design improvements than might otherwise be considered.
For a more structured approach, you can design your study from the bottom up to directly assess key SDT measures, such as hit rates and false alarm rates:
Hit rate (H): Measures how often users correctly identify a true signal. For instance, if users correctly identify 8 out of 10 alerts, the hit rate is 80%. This provides insight into how effectively your design is communicating vital information.
False alarm rate (F): Measures how often users incorrectly identify a signal when none is present. For example, if 2 out of 10 non-urgent alerts are mistakenly flagged as urgent, the false alarm rate is 20%. This helps to identify and reduce unnecessary disruptions caused by false positives.
The goal at this level isn’t necessarily statistical certainty, but rather a richer classification of user errors than standard task success rates. For instance, if you’re testing new payment options in an e-commerce checkout process, you might structure tasks to see how well users identify them, and calculate hit and false alarm rates to assess their effectiveness. Developing the study in such a way that you can collect these measures can not only guide design recommendations, but provides a baseline for comparison with future iterations.
Some researchers will have had experience conducting formal SDT studies in academic labs, often in a tightly-controlled environment with many trials for statistical testing. While this level of rigor is excessive for most UX projects, certain high-stakes scenarios might benefit—such as those involving medical devices, for example. In such cases, consider the following advanced measures:
Sensitivity (d’): Quantifies how well users can distinguish between signals and noise. Higher d’ values indicate better discrimination, while a d’ of zero means the user cannot distinguish between the two. For example, you might use this as an aggregate measure to measure how effectively representative users in a study distinguish alerts.
Criterion (c): Reflects the threshold a user sets for deciding whether something is a signal or noise. A lower criterion might lead to more hits but also more false alarms, whereas a higher criterion would result in fewer hits and fewer false alarms. Understanding this helps explain why different participants respond differently to the same interface.
Response bias (β): Measures the user’s tendency to favor one response over another. A β value of 1 indicates no bias, while values below 1 suggest a bias towards saying “yes, there’s a signal,” and values above 1 indicate a bias towards saying “no.” This helps to understand individual differences in overall decision-making strategies.
These measures are interconnected—changing one affects the others. For instance, a user with a low response bias (more likely to say “yes”) may also have a lower criterion, which may well manifest in lower sensitivity.
Imagine your team has been tasked with testing a medical device for diabetic patients that requires users to detect changes in insulin levels. You could design a formal experiment, calculating outcomes such as sensitivity (d’) to understand how effectively users detect critical changes and precisely which design elements influence their sensitivity. This level of rigor gives product teams confidence that their designs will improve user outcomes and reduce organizational risk.
In most UX research studies, incorporating SDT will typically involve using hit rates and false alarm rates within the study design, or simply applying it as a conceptual lens to interpret the data. Few studies will require the caution of a multi-trial lab experiment. But whether you apply SDT conceptually or through detailed measurement, you can gain a deeper understanding of how users differentiate between signals and noise in your designs.
The bottom line
Users often make an uncertain judgment about whether the information they need is present or absent. In this article, we’ve explored signal detection theory as a powerful approach to better understand these decisions, and:
We examined a matrix comparing judgments about signals and their actual presence, which categorizes user decisions into hits, misses, false alarms, and correct rejections.
We looked at real-world applications of this framework, from TSA screenings and phishing detection to identifying misinformation and evaluating product details pages.
We also discussed the different levels at which SDT may be applied in UX research: at a conceptual level or by including explicit measures from SDT, or in rare cases through formal lab experiments.
Additionally, we reviewed the key outcome measures of SDT, including hit rate, false alarm rate, sensitivity, response bias, and criterion—tools that provide different insights about the user decision-making processes.
Traditional task success rates are versatile, but they can leave us with unanswered questions about the reasons behind a user’s failure to achieve their goals. In some situations, SDT can fill in the gaps, offering a fuller understanding of how users perceive and interpret information within an interface.
With this understanding of SDT and its applications, consider when it may be appropriate to apply it in a user study. By leveraging SDT, you’ll gain clearer insights into the judgments users make, enabling you to design interfaces that not only meet their needs but also guide them effectively through the noise to the information they seek.
Drill deeper…
Depth is produced by Drill Bit Labs, a consulting firm on a mission to advance the field of user research. We partner with leaders in UX and digital product development through research projects, education and training, and strategic advisory support.
These services help make all our free industry reports and content possible. We’d be happy to open a conversation to discuss your specific needs and goals for this year.
Further resources
Mak Pryor’s case study using SDT as part of an ecommerce usability evaluation
Stanislaw and Todorov’s 1999 paper on calculating common SDT measures
R package for computing coherence, sensitivity, and others
Coming up
Later this month, we’ll resume our series producing a company-agnostic, data-driven career ladder for UX Researchers, based on analysis of 1,394 job descriptions collected over a period of months. The first article discussed what we can learn about UXR roles from job post metadata, including popular titles, seniority levels, and salaries. Next up, we’ll look at findings from the qualifications sections of job posts, including education and years of experience.